LeafLineage

The purpose of this tool is to provide a quick overview of the main concepts (dictionary of features) employed by Extant Leaves classification model for each of the 142 Leaf classes, along with an associated importance score for these concepts. For instance, when classifying "Betulaceae" the model utilizes features such as Stem, Leaf Blade + Midrib, Leaf Blade. Click on some examples to get started or use the search field at the top to find a specific class.
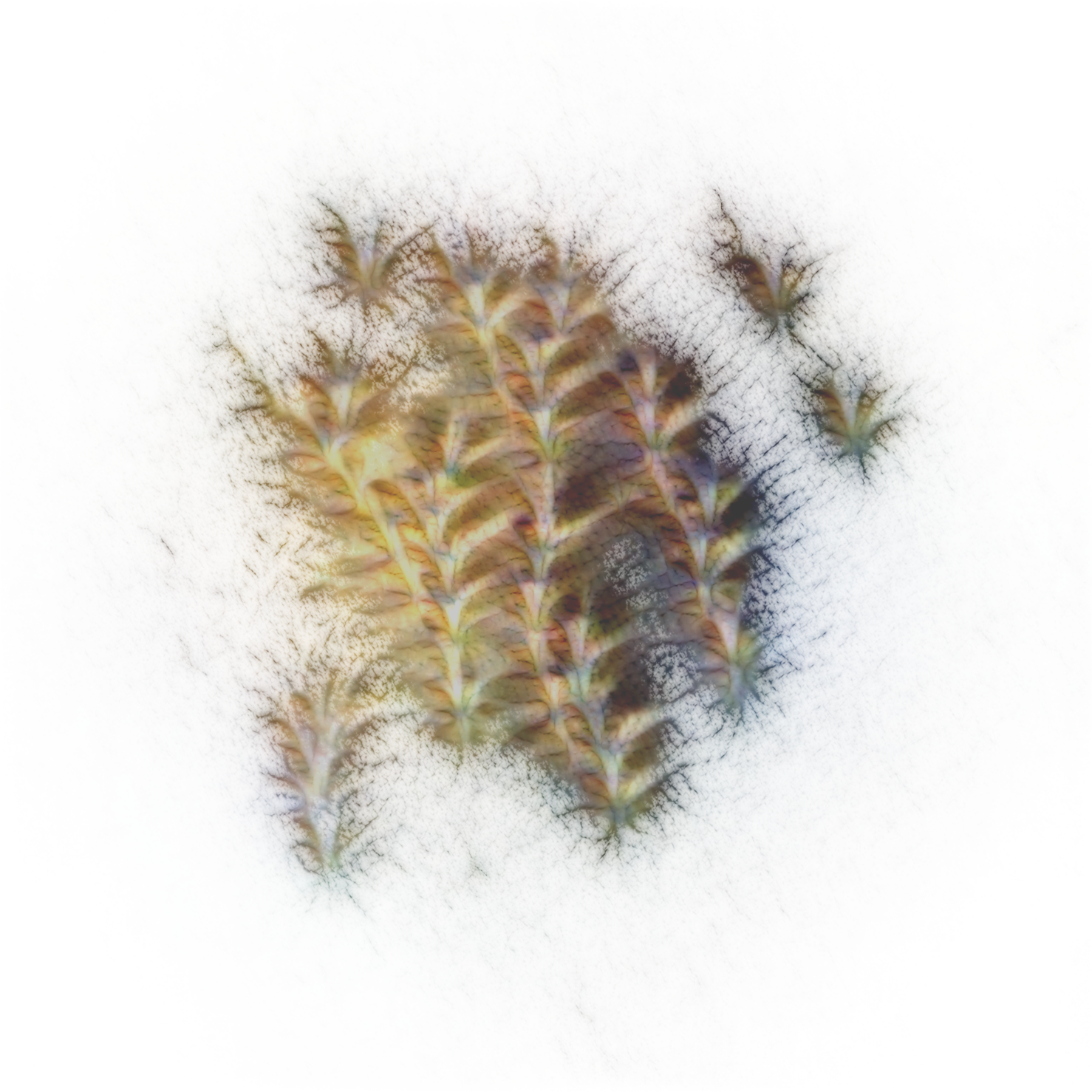
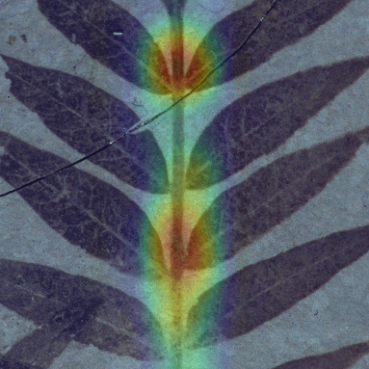
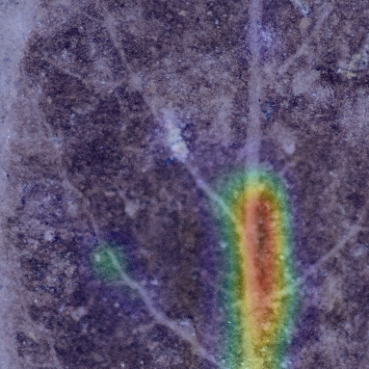
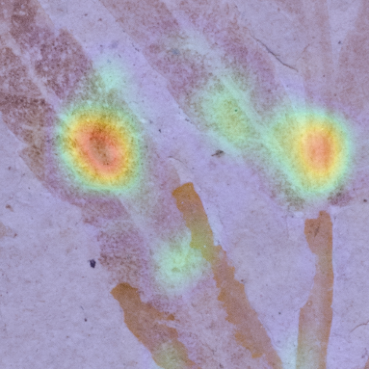
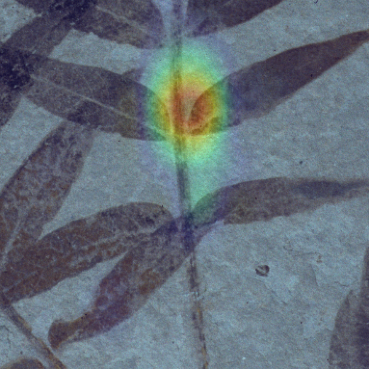
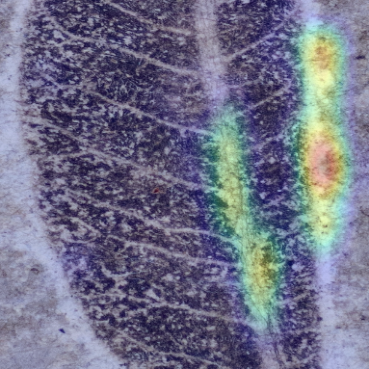
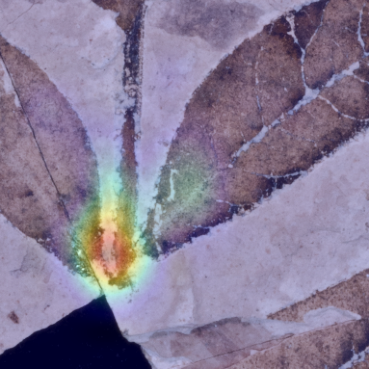
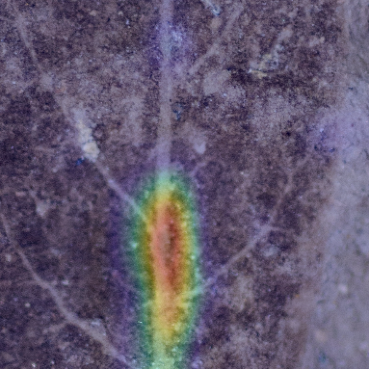
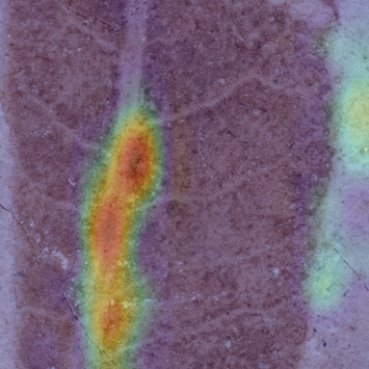
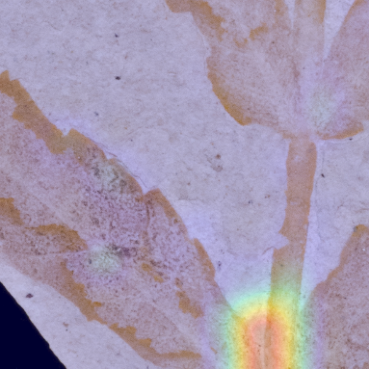
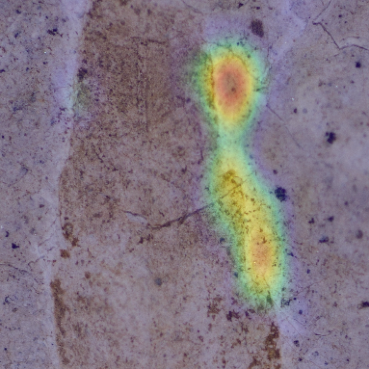

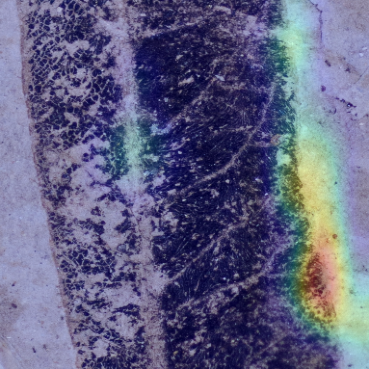
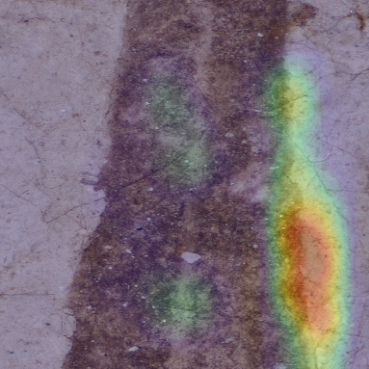
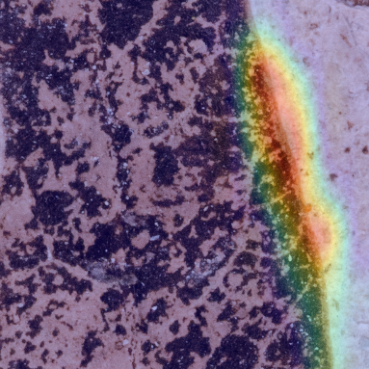
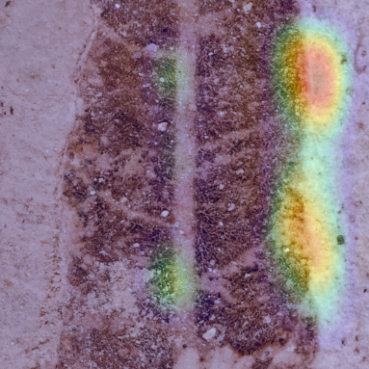
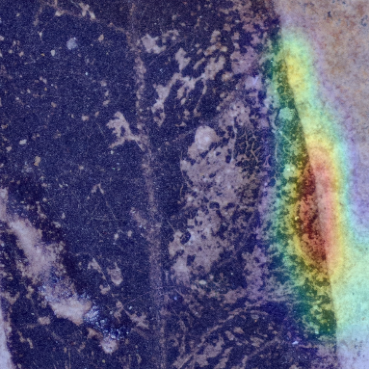
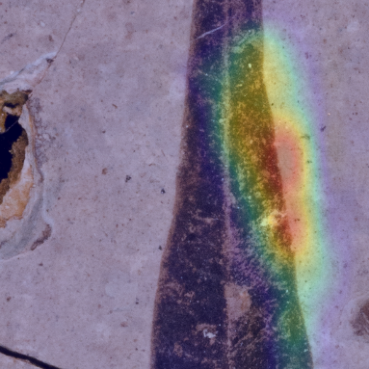
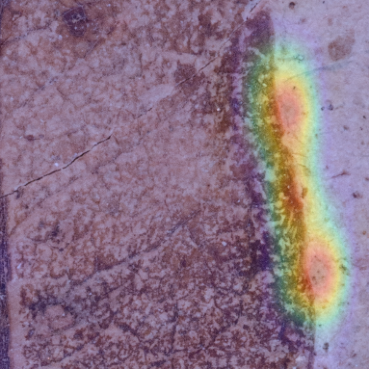
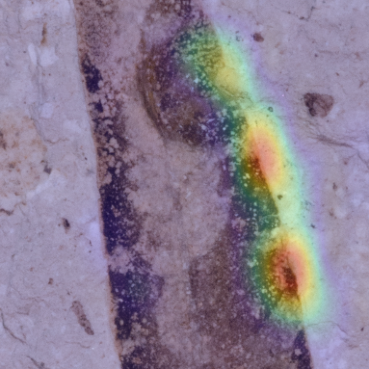
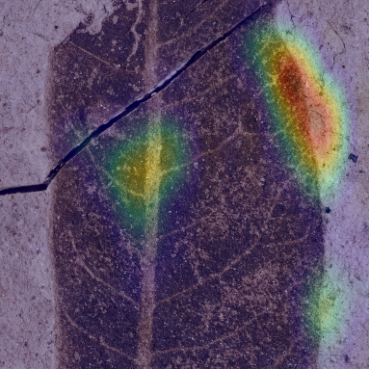
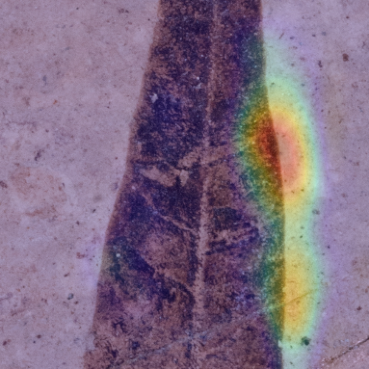

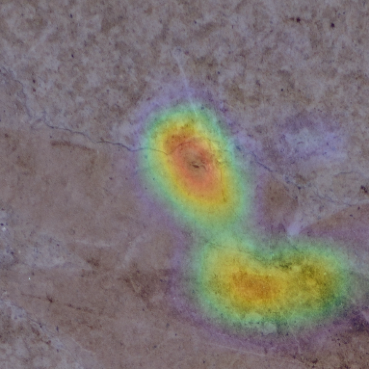
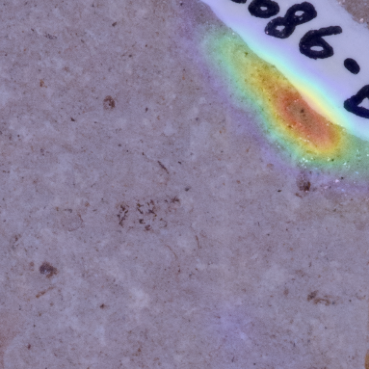
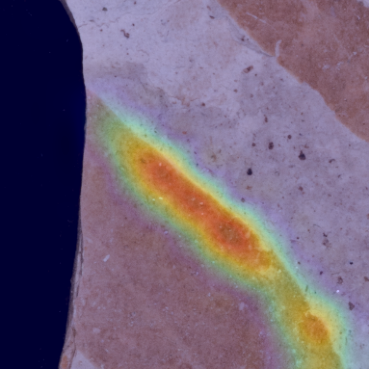
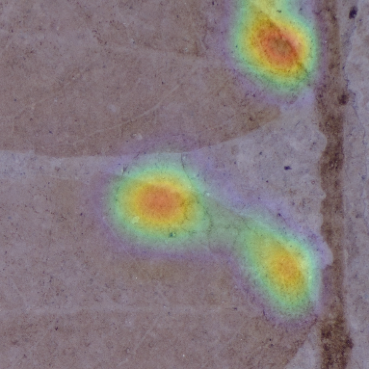
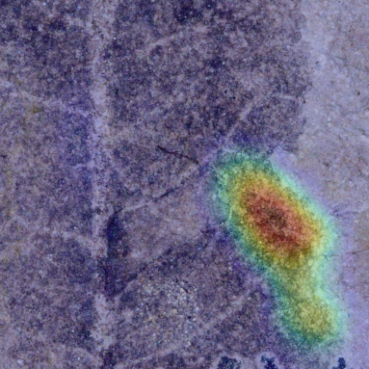
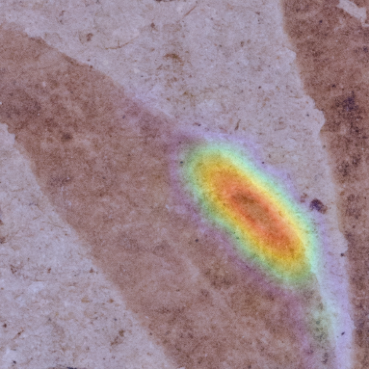
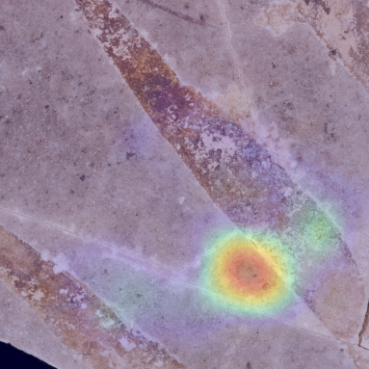
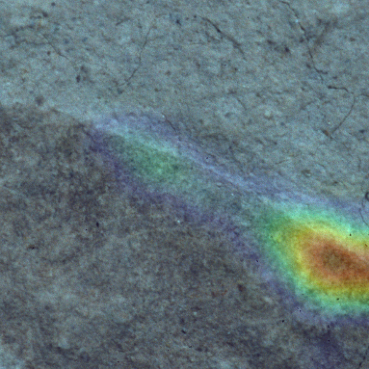
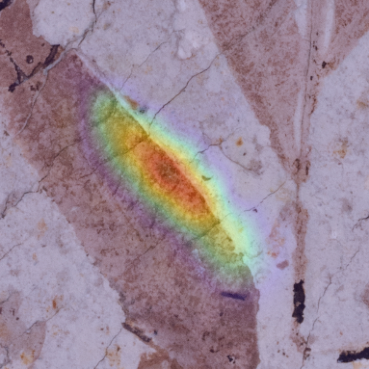
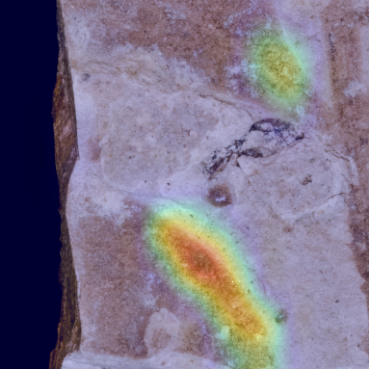

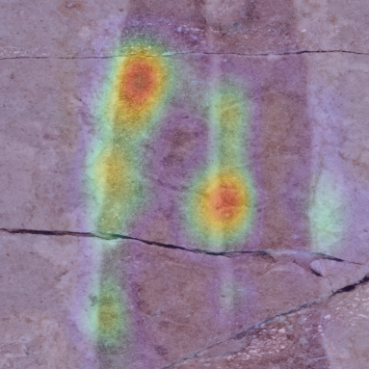
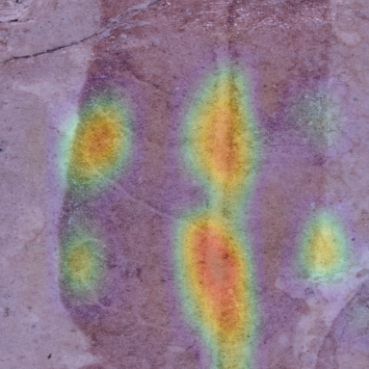
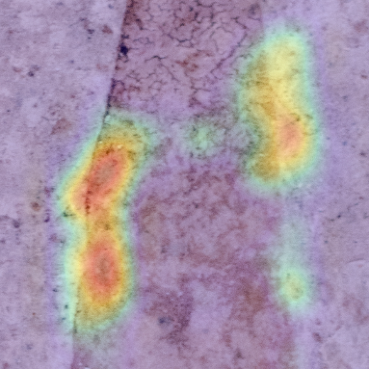
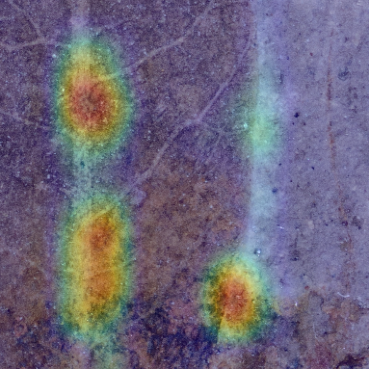
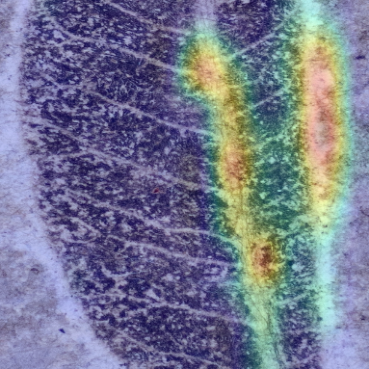
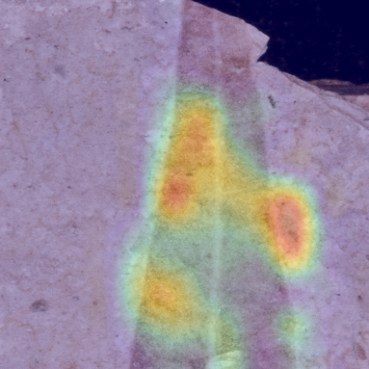
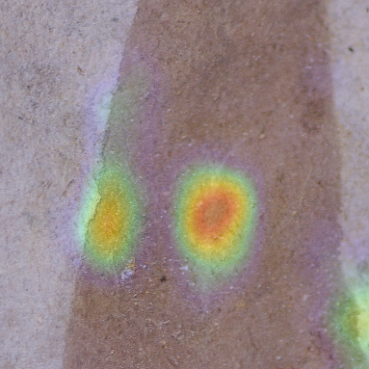
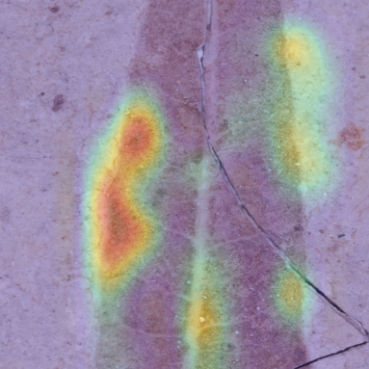
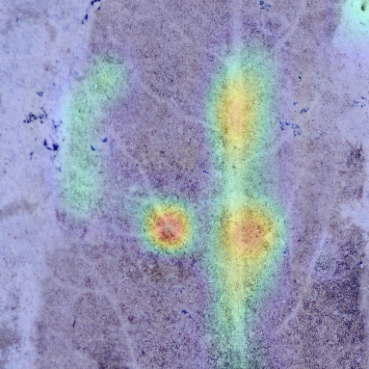
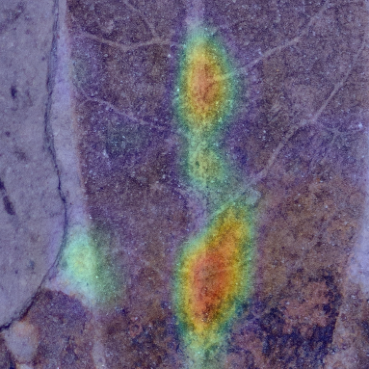
How does it work?
LENS is straightforward: it's a method that automatically (they are not predefined) retrieves the concepts (or visual atoms) used by a model for a given class, and assigns an importance score to each of these concepts. The website allows scrolling through the top 10 most important concepts for each class. Each concept is represented by a feature visualization, meaning an image that exemplify the concept (or epitomizes it). By clicking on this feature viz , users can view crops of images that activate this concept. Keep in mind, the higher the importance score, the more determinant the concept is for the model.
You can start exploring a class by clicking on one of the feature viz below:




Alternatively, you can begin exploring on your own using the search engine (at the top) or by clicking the
.🗞️ Citation¶
If you are using LENS as part of your workflow in a scientific publication, please consider citing one of the articles we build on:
@inproceedings{fel2023craft,
title = {CRAFT: Concept Recursive Activation FacTorization for Explainability},
author = {Thomas Fel and Agustin Picard and Louis Bethune and Thibaut Boissin
and David Vigouroux and Julien Colin and Rémi Cadène and Thomas Serre},
year = {2023},
booktitle = {Proceedings of the IEEE Conference on Computer Vision and
Pattern Recognition (CVPR)},
}
@article{fel2023holistic,
title = {A Holistic Approach to Unifying Automatic Concept Extraction
and Concept Importance Estimation},
author = {Thomas Fel and Victor Boutin and Mazda Moayeri and Rémi Cadène and Louis Bethune
and Léo andéol and Mathieu Chalvidal and Thomas Serre},
journal = {Advances in Neural Information Processing Systems (NeurIPS)},
year = {2023}
}
@article{fel2023unlocking,
title = {Unlocking Feature Visualization for Deeper Networks with
MAgnitude Constrained Optimization},
author = {Thomas Fel and Thibaut Boissin and Victor Boutin and Agustin Picard and
Paul Novello and Julien Colin and Drew Linsley and Tom Rousseau and
Rémi Cadène and Laurent Gardes, Thomas Serre},
journal = {Advances in Neural Information Processing Systems (NeurIPS)},
year = {2023}
}
📝 License¶
The project is released under MIT license.
-
Learning a Dictionary of Shape-Components in Visual Cortex: Comparison with Neurons, Humans and Machines ↩
-
Interpretability Beyond Feature Attribution: Quantitative Testing with Concept Activation Vectors (TCAV) (2018). ↩
-
Towards Automatic Concept-based Explanations (ACE) (2019). ↩
-
Invertible Concept-based Explanations for CNN Models with Non-negative Concept Activation Vectors (2021). ↩
-
Thread: Circuits: What can we learn if we invest heavily in reverse engineering a single neural network? (2020). ↩
-
Feature Visualization: How neural networks build up their understanding of images (2017). ↩
-
Progress measures for Grokking via Mechanistic Interpretability (2023). ↩
-
Which explanation should i choose? a function approximation perspective to characterizing post hoc explanations. (2022). ↩
-
Towards the Unification and Robustness of Perturbation and Gradient Based Explanations. (2021). ↩
-
Towards Faithfully Interpretable NLP Systems: How Should We Define and Evaluate Faithfulness? (2020). ↩
-
Interpretable Explanations of Black Boxes by Meaningful Perturbation. (2017). ↩
-
On the (In)fidelity and Sensitivity of Explanations. (2019). ↩
-
Evaluating and Aggregating Feature-based Model Explanation. (2020). ↩